Challenges to Traditional Fraud Detection
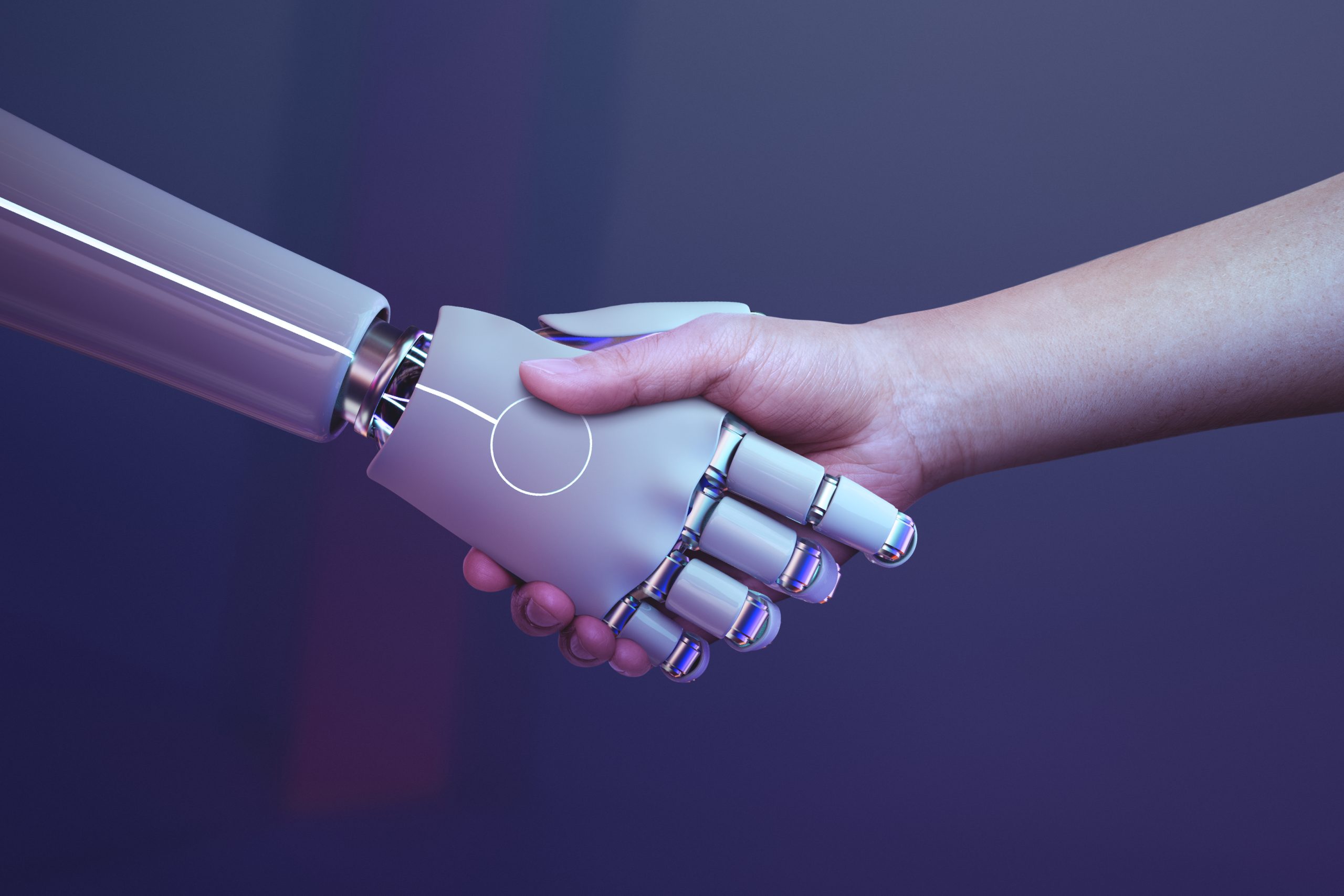
- Detection isn’t real-time, so fraud is detected after a financial loss occurs.
- Rules are binary and limited. They don’t accommodate the complexity and combinations of input variables that can be evaluated. This limitation results in high numbers of false positives.
- Rules are hardcoded into business logic. Curating the rules, incorporating new data sources, or adding new fraud patterns usually requires application changes that affect a business process. Propagating changes throughout a business process can be cumbersome and expensive.
- Businesses must both efficiently authenticate customers to confirm they are who they claim to be and safeguard their operations against fraud.
- Consumers who weren’t already digitally knowledgeable may find it challenging to distinguish between a legitimate engagement with an organization and a potential scam as a result of the process that comes with moving to digital.
- Consumers expect quick and easy digital journeys, but they also expect organizations to prioritize the security of their information.
- For businesses, maintaining a review of their current client risk profiles is expensive. Not just at the application stage, but throughout the customer journey
Benefits
Win customer trust: online security is a top concern for most of the consumers. By successfully securing their digital experiences can win consumer trust and gain access to a wider range of data to personalize the customer relationship at every touchpoint.
Move fast and prevent losses: Move from rules to machine learning to respond in time and reduce operational costs associated with fraud
Scale flexibly: Cut through all the data complexities and implement fraud prevention at scale with the Data lake and Data Vaults.
Faster and more efficient detection: The system gets to quickly identify suspicious patterns and behaviors that might have taken human agents months to establish.
Reduced manual review time: The amount of time spent on manually reviewing information can be drastically reduced when you let machines analyze all the data points for you.
Better predictions with large datasets: A machine learning engine gets better trained the more data you feed it. Consequently, while enormous datasets can sometimes make it difficult for humans to detect patterns, this is actually the opposite with an AI-driven system.
Cost-effective solution: You only need one machine-learning system to process all the data you put at it, regardless of volume, as opposed to adding more RiskOps agents. This is perfect for companies who see seasonal fluctuations in traffic, checkouts, or signups. A machine learning system can help your business grow without significantly raising risk management expenses concurrently.